‘All happy molecules are alike; each unhappy molecule is unhappy in its own way.’
Anna Karenina Principle, adapted from Tolstoy
For a drug to be useful for treating a disease, it ‘only’ needs to fulfill two scientific criteria (we leave out commercial aspects here): It needs to be efficacious (improve disease state, which may also be symptomatic, at least for a subset of the patient population, and usually compared to standard of care); and this needs to happen at sufficient safety (or tolerable toxicity, which of course is in turn related to effective dose and indication).
Both of those aspects seem superficially mirror images of each other, but far from it – efficacy is the presence of one desirable property, of which there may be one or more which are leading to the desired effect, such as up- or downregulating a particular biological pathway (or many other things). On the other hand, safety is the absence of a long list of undesirable properties, which by its very nature is more difficult to deal with in practice.
So both parts are fundamentally different in nature – efficacy is the selection for the presence one given property (which realistically can only be defined on an organism/in vivo level). Safety means absence of many possible events, and this by its very nature is very difficult to predict. How do you predict an unknown mode of toxicity that you haven’t seen before? Or the potential toxicity of an entirely novel structure?
Visually we can represent this as follows (based on a drug approved in 2019, the first one to treat postpartum depression, brexanolone):
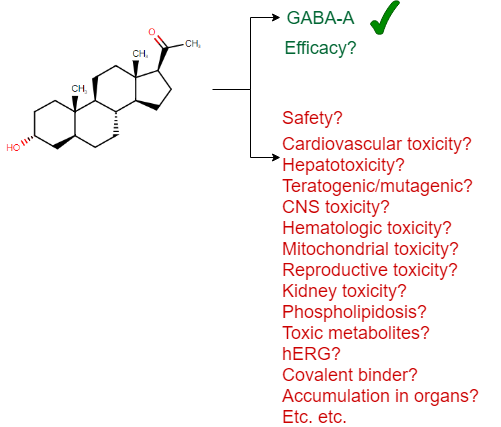
(I should add that there is no claim that this particular drug is unsafe in any way – it is simply a recently approved drug which has been used here as an example for the many aspects of safety one needs to consider, and I could have picked any other example as well to underline this general principle.)
This is also what makes developing a drug so much more difficult than ‘finding a ligand’ (which in particular in academic publications is often equated with ‘drug discovery’) – it’s the in vivo behaviour that counts, and a whole balance of phenotypic endpoints. This is why we have millions of ligands in ChEMBL, but only a few thousand approved drugs – many compounds are active on a target, some are even achieving an efficacy-related endpoint; but tolerable safety thins out the field considerably.
Toxicity may also be either mechanism-related, or not mechanism related, which provides a very different context – eg early anti-cancer drugs with unspecific cytotoxicity could easily assumed to have mechanism-related toxic effects. However, the non-mechanism related toxicities are more difficult to anticipate, since one doesn’t initially know what to look for.
Given the interest in ‘AI’, also the field of In silico toxicology has received considerably more attention in the recent past. So where do things stand currently – and how good are we at using computational algorithms for predicting the toxicity of small molecules? This is what this post is aiming to summarize. (By its nature it will be rather brief though, for more details the reader is referred to recent reviews on databases and approaches in the field.)
In the following four approaches for toxicity prediction will be distinguished, which are based on different types of data:
- Target/Protein-based toxicity prediction (analogous to target-based drug discovery), which also forms the basis of eg safety pharmacology, and which is hence regularly employed in practice: You screen a compound against a dozen (or up to a hundred) proteins, and hope that the results will be informative. The ‘target’ of a drug may be also related to efficacy at the same time (‘on-target related toxicity‘), or it may be an ‘off’-target related toxicity, but in any case there is some link assumed to be present between ligand activity against a protein, and a toxic effect of the compound. Basically, this part of safety assessment means: ‘We know where we are in bioactivity space, but we don’t quite now what this means for the in vivo situation‘ (how relevant the target really is, see below);
- Biological readout (‘omics’) based toxicity prediction, eg based on transcriptomics (‘toxicogenomics‘), imaging data, or other high-dimensional readouts derived from a more complex biological system than activity against an individual target. In this case, biological readouts are not on the target (direct ligand interactor) levels, but they can be enumerated (genes, image-based readout variables), and some features may be more directly interpretable (genes), while others are less interpretable (morphological features). This corresponds to the situation ‘We are capturing biology on a large scale, along one or few readout types, and hope that those readouts tell us something relevant for toxicity’ – which always needs to be shown. I have learned about this in the context of analyzing high-content screening data which was generated in a hypothesis-free manner and where interpretation and use of data was far from trivial);
- Empirical/phenotypic toxicity – In this case toxicities are measured ‘directly’ in a ‘relevant model system’, say the formation of micronulei, hepatotoxicity etc. In this case (usually, but not always) cellular effects are, as opposed to the previous point, not mapped to annotated genes/pathways, and the toxicity/empirical endpoint derived from the system is taken as the readout variable. This represents the situation ‘We don’t really know where we are in bioactivity space and in biology, but we are more confident that the readout that is relevant for compound toxicity in vivo’; and
- Systems models – Conceptually different from the above four categories, ‘systems models’, either on the experimental or computational level, are aiming to model complex biological systems, such as ‘organs on a chip‘, or by using metabolic network models or cellular signalling models. They can conceptually be used for any aspect of understanding and predicting compound action in vivo (both related to efficacy and toxicity). While this category of models may not be fully functional in many areas currently, I have big hope for the future, given they may well represent useful trade-off between complexity and cost (ie, practically useful predictivity at reasonable cost).
So to summarize, the current types of data we can use for in silico toxicity prediction are visualized as follows:

The level of abstraction, and understanding of the system, need to be related to choose meaningful readout parameters. Relevance for the in vivo situation then depends on the strength of the link between readouts generated and their impact in man, as well as quantitative aspects such as PK/exposure (in addition to practical assay setup questions, see below). Note that this is a conceptual figure, and details differ depending on readout, and the particular application area (drug discovery vs consumer safety etc.)
So how well in ‘AI’/In Silico Toxicology doing today, in the above four areas? Where can we predict safety, or toxicity, sufficiently well?
1. Target-based toxicity aims to use on-target activities as predictors to anticipate toxicity. This is an appealing concept due to its simplicity (protein assays are easy to perform generally), hence its frequent use in safety profiling in pharmaceutical companies. However, when it comes to its relevance to the in vivo situation, and hence practical relevance, two fundamental questions exist:
- What is link between activities against protein targets, and adverse reactions observed in vivo? Some recent reviews exist which link the two (Bowes et al., Lynch et al., Whitebread et al.), and ever since working as a postdoc at Novartis on links between protein targets and adverse reactions years ago I wondered who picks proteins to be measured in safety panels, and using which types of criteria. However, quantitative information between protein activity and adverse reactions caused is hard to obtain, due to many reasons (the biases in reporting drug adverse reactions being a particularly big contributor here, which has been the basis of some recent studies as well). If we look at the available data from our own analysis, based on relationships between protein activities and adverse reactions observed of marketed drugs, another problem emerges though (which is the result of work by Ines Smit in my lab, publication is currently under preparation):
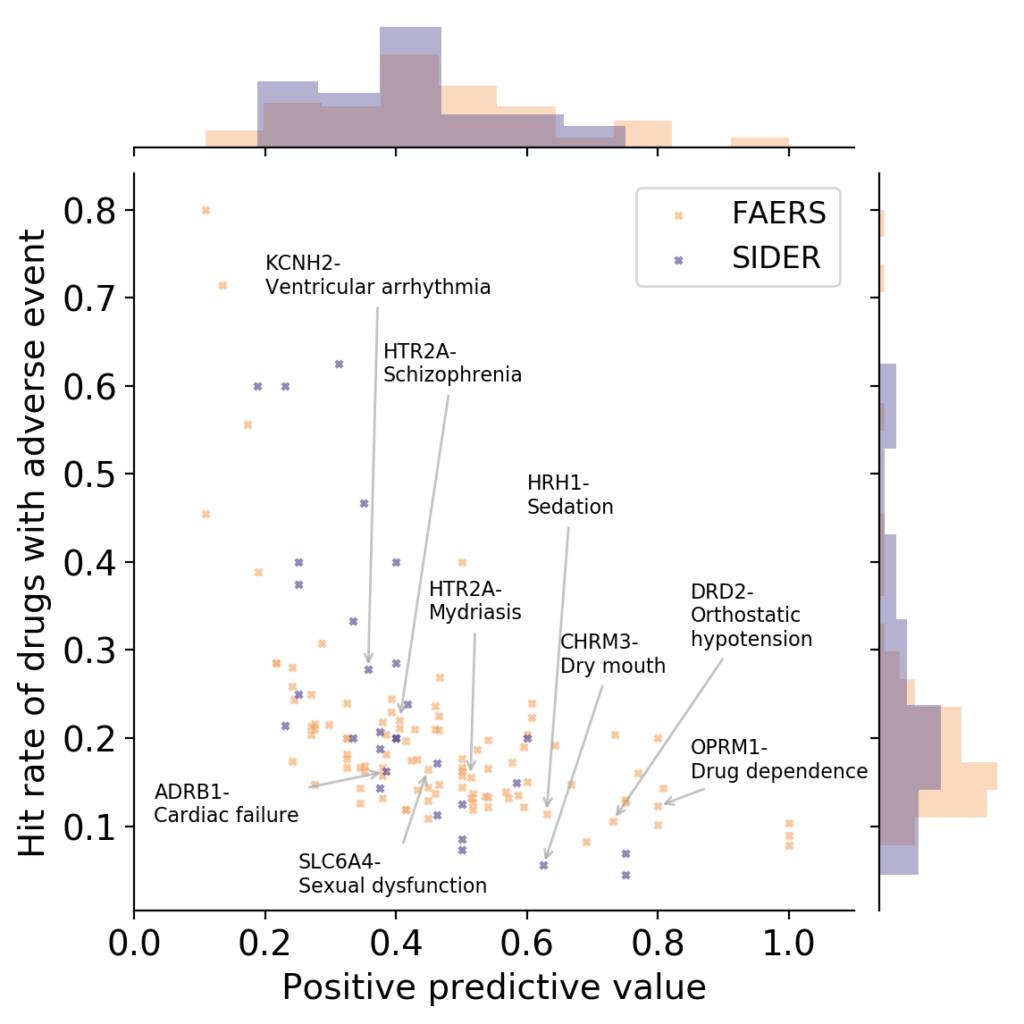
OPRM1: Mu opioid receptor, HTR2A: Serotonin 2a (5-HT2a) receptor, DRD2: Dopamine D2 receptor, SLC6A4: Serotonin transporter, HRH1: Histamine H1 receptor, ADRB1: Beta-1 adrenergic receptor, CHRM3: Muscarinic acetylcholine receptor M3, KCNH2: HERG/Potassium voltage-gated channel subfamily H member 2 (Work by Ines Smit, to be published shortly)
It can be seen in the above figure that some proteins are rather good at detecting adverse events (high positive predictive value), but they do so at low hit rates (low sensitivity; bottom/right). Or you have proteins with high hit rate (sensitivity), but they have low positive predictive values (ie, in many cases activity against a protein does not lead to an adverse event; top/left). So – choose your devil! Quite possibly combinations of assays perform better such as rules learned on assay data, but the main point remains that protein-to-adverse reaction mappings are no simple 1:1 relationships, far from it. (I should add that the positive predictive values listed above are significantly larger than those one would expect by random, so there certainly is some signal in them. However, the key question is: how can we use this information for decision making, instead of just creating a ‘worrying machine’ that throws up warning signs all the time? For this we still need to understand better which information is contained in particular assay readouts, in their combinations, and how this translates to the human situation.)
The other big problem of course is in vivo relevance, due to compound PK and exposure. What is the route of administration, metabolism, excretion etc. associated with a compound? We often simply don’t know (though efforts such as the High-Throughput Toxicokinetics Package try to bridge that gap). However, given that ‘the dose makes the poison’ this is something we need to know in order to make an informed decision.
Hence, target-based ways of anticipating safety have severe limitations. In the context of ‘AI’ and computational methods this is even more of a limitation since this basically the only area where we have sufficient amounts of data (target-based activity data), but for in vivo relevant toxicity prediction this is often simply not good enough – we don’t really know what a prediction in this space means for the in vivo situation.
One might argue that at least for some proteins a link between in vitro effects and adverse reactions is clearly given, such as evidenced by the focus say on hERG activity and its links to arrhythmia in the last 15 years or so. However, this seems often be driven by the need for simple testable endpoints, rather than extremely strong in vitro–in vivo links, as more recent studies made clear.
So to summarize, when it comes to protein activities we have (relatively speaking) lots of data, of tolerable quality, but the relevance for the in vivo situation is not always warranted. This is due to unknown links between protein targets and adverse drug reactions, but also due to the immense uncertainty of quantitatively extrapolating in vitro readouts at a defined compound dose to the in vivo situation with often less defined PK and exposure.
2. Biological readout-based toxicity prediction – In this case, biological, high-dimensional readout are employed for the prediction of toxicity, in practice (mostly due to the ability to generate such data) often gene expression data, or more recently also imaging data. We have some experience in this area ourselves, such as in the QSTAR project with Janssen Pharmaceuticals, which aimed to use gene expression data in lead optimization. There are obviously also studies from other groups around, aiming eg to predict histopathology readouts based on gene expression data. In line with the generally increasing interest in ‘-omics’ data and high-dimensional biological readouts, also the EPA proposes to follow this route in their ‘Next Generation Blueprint of Computational Toxicology at the U.S. Environmental Protection Agency‘, and to use both gene expression and imaging data for profiling of chemicals, to somewhat adjust their earlier efforts with the ToxCast profiling datasets. And I do think they have a good point: Biological readouts create for relatively little cost (depending on the precise technology chosen) a lot of data, meaning they profile compound effects rather broadly (for not just one endpoints, but everything that can be measured, under given assay conditions, along the given slice of biology considered).
The question is, though: Is this also relevant for the prediction of toxicity? Is there a signal – and if so, where is it?
This is a tricky question to answer – a very tricky question actually, when one is trying to generate practically useful models for toxicity prediction based on -omics readouts. Consider that only some of the variables that need to be considered in this case are as follows:
- What is the dose of a compound to be tested? (At low dose there is often no signal, at high dose this is not relevant for the in vivo situation! Also, identical concentrations are not actually ‘the same’, since eg drugs are given at different doses, and show different PK, so there is no objective way to choose consistent assay conditions). Should a compound be given at single dose, or repeat dosing? What is relevant in humans?
- What is the time point to be chosen for readouts? Early stage (eg 6 hours), or later stage (eg 24 or 48 hours)? How is this related to later dosing of the drug (which of course is often not known early on)?
- What is the precise biological setup to be used, eg the cell line (or better spheroids, co-cultures etc)? Is the signal from one cell line relevant for others (and what is actually the distribution of the compound in vivo, across tissues and cells…)?
- How do we normalize data – should we use housekeeping genes (or are those not so stably expressed after all)?
- How is toxicity to be defined? In vivo endpoints are noisy and variable (but tend to be more relevant); lower-level endpoints (say, cellular cytotoxicity) are often less noisy (but tend to be also less relevant). If using eg histopathology data, do we use single endpoints or combinations thereof? (How can we summarize data? Are there actually ‘classes’ of toxicity, which we need for model training?)
- How do we deal with variability between eg animal histopathology readouts? Control animals that show significant histopathology (which always happens in practice)?
- Etc.
So as we can see, just ‘generating lots of data’ will not do – we need to generate relevant data. What this means though, depends on the case (compound etc.)
Generally I believe using biological high-dimensional readouts is worthwhile, since one samples large biological space at often a suitable cost. We use it a lot as well – eg for repurposing using gene expression data works rather well. However, on the one hand this relates to efficacy, not toxicity, which is hence very different in nature (see above). On the other hand it is unclear how to define the biological setup of the system where data is measured. (One could even claim that either one has a consistent setup, or one that is relevant for the in vivo situation, but not both at the same time…), how to define toxic endpoints, and how hence to generate sufficient data for such models. This is at odds with some of the literature from the ‘AI’ field which eg happily discuss toxicity prediction with RNA-Seq data, but which signal in such data predicts precisely which in vivo relevant endpoint is not really clear to me, and I think this needs to be worked out in much more detail to be practically useful.
So while technical problem are partially solved to generate biological high-dimensional readouts, practical aspects of how to generate such data, and which signal is predictive for which toxic endpoints seems, on the whole, still largely work in progress. Inconsistently generated data will lead to problems when training computational toxicology models though.
3. Empirical/phenotypic toxicity – in this case we have a model system to generate empirical toxicity readouts for compounds. Hence, this type of data is on the one hand based on a complex biological system (certainly more complex than isolated proteins); but on the other hand we do not care about individual biological parameters (be it binding to a receptor, or the up- and downregulation of a large number of genes). Instead, we rather link a compound structure to an empirical, phenotypic endpoint. Eg the micronucleus assay might be one such type of assay, animal toxicity studies which consider particular endpoints might be another. There has been lots of discussion on the predictivity of animal studies, generally concluding that in many cases, depending on animal chosen and toxic endpoint considered, animals often indicate the presence of toxicity (ie, a compound toxic in animals is often toxic in humans); while they are less good at indicating the absence of toxicity (ie, a compound not toxic in animals is not necessarily safe in humans). See eg Bailey, as well as the reply to the work and related publications for a more detailed discussion.
What is the problem with this group of endpoints in the context of in silico toxicity prediction? On the positive side the endpoint is, if well-chosen, relevant for the in vivo situation, which is a big plus already (see comments above related to target-based data, and biological readouts as a contrast). On the other hand, depending on the particular assay, we might still not have suitable translation to in vivo situations, mostly due to lack of estimating PK/exposure properly. In addition, as mentioned in the introduction, empirical toxicity readouts only consider what is explicitly examined – we only see the histopathological readouts we examine, we only detect the toxicity that we set up an assay to detect. But, since the list of possible toxicities a compound may have, is very large, the coverage of toxicity space is difficult to achieve. (I will leave out a more detailed discussion of using animal-based readouts for safety analysis at this point, which opens up a whole new set of questions due to the much more complex system used.)
That being said, one area of toxicology, namely (digital) pathology, with the ability to classify readouts more quickly and more consistently than humans has benefited greatly from the advent of machine learning. This area is basically made for applying Convolutional Neural Networks (CNNs) with its performance in image recognition, although in some cases additional technical steps need to be taken to address eg the rotational invariance of cellular systems. While this addresses some issues related to consistency of data, the questions how to extrapolate from animal histopathology to human relevance of course remains, in addition to the question how to define toxic endpoints in a relevant manner (based on which readouts and quantitative thresholds).
So what does this mean for predicting toxicity in silico, based on empirically measured toxicity data? We measure in systems which may at least partially be more representative of organisms, which is a plus. On the other hand, translating in vitro results (or those from animals) to the in vivo human situation is difficult due to often little understanding of PK of a new compounds, and also conceptually it requires an enumeration of explicitly chosen toxic endpoints – we only see what we explicitly look for.
4. Whole systems modelling – As opposed to the above three approaches, modelling a whole biological system, either in a simplified experimental or in a computational format, aims to combine both practical relevance of the readout or model, as well as being fast and cheap enough to be used practically to make decisions. This can mean experimentally eg the ‘organ on a chip models‘, or computationally cellular models or virtual organs such as intestines or the heart. Some of those models, such as the organ on a chip model linked above, have achieved remarkable things, such as ‘to establish a system for in vitro microfluidic ADME profiling and repeated dose systemic toxicity testing of drug candidates over 28 days’.
Simple models (such as activity against protein targets) often have little predictivity for the in vivo situation, and we are unable to exhaustively test all (or sufficient) chemical space in all (relevant) empirical tox models. Therefore, either experimentally or computationally staying at level that is biologically sufficiently complex to be predictive and relevant, but at the same time practically applicable (with respect to time and cost), seems an appropriate thing to do. The question for this class of models is to what extent biology can be reduced to stay representative – be it by identifying which cells or cell systems, in which arrangement, need to be present to interact to be predictive; or computationally which (eg on the cellular level) genes, and interactions, need to be present to achieve this goal.
What hence remains to be done is to establish which parts are needed to model the system, and how to parameterize the experiment, be it truly experimental (cellular setup, etc.), or in silico (eg on the cellular level parameterizing interactions between genes etc.) which is no trivial exercise. Subsequently, such systems of course need to be validated properly – which has been done in some cases, eg in case of the heart, with remarkable success, where “Human In Silico Drug Trials Demonstrate Higher Accuracy than Animal Models in Predicting Clinical Pro-Arrhythmic Cardiotoxicity“.
Hence, while whole systems models are – generally speaking – probably still in their infancy, both from the experimental and computational point of view, it appears to me that this will quite possibly be a suitable way to have both practically relevant and applicable models available for in silico toxicity prediction in the more distant future. This is due to the fact that systems try to resemble in vivo situation at suitable biological complexity. The problem is that it will still take a while to validate such models, and likely consortia are needed to generate data for in silico models on a sufficient scale, so this will likely not happen tomorrow in all areas.
So where do things stand now for ‘AI’/In Silico drug toxicity prediction?
Currently the situation can be summarized in a way that we didn’t yet find a suitable biologically relevant abstraction of the system to generate sufficient amounts of data for many relevant toxic endpoints, and we are not yet properly able to extrapolate from model-based readouts to the in vivo situation (due to PK/exposure), though some steps such as the HTTK package of course points into that direction.
The following gives a table of which types of data can be used for in silico toxicology, and what their advantage and disadvantages are:
Toxicity Prediction based on… | Advantages | Disadvantages |
Protein-Based Bioactivity Data | Easy to generate Lots of data | Does not consider exposure/PK (in vivo relevance unclear) Often either low sensitivity or positive predictive value for clinical adverse reactions |
Biological readouts (gene expression, images, …) | Considers complexity of biologoical system Broad readout | Setup for in vivo relevance not trivial (dose, time point, cell line, …) Defining ‘toxic’ endpoints not trivial |
Empirical toxicity modelling | Potentially in vivo relevant endpoint can be replicated in assay | Translation (PK/exposure) often unclear Needs explicit enumeration of toxic events |
Systems Approaches | Consider whole system Combine suitable level of complexity/predictivity and manageable cost | Currently in their infancy Extensive parametrization necessary |
Hence, we either have large amounts of less relevant data for the in vivo situation (eg target based profiling data); medium amounts of likely more relevant but also hugely complex data (biological readouts) where both generating and finding a signal in the data is not trivial; or very specific data for individual types of toxicities. This, naturally, makes the construction of reliable in silico toxicity models difficult at the current stage. (Though this should not be an oversimplification here – there may very well be suitable computational models available in areas where it was possible identify a suitable model system to generate sufficient amounts of relevant data, but I would doubt this is currently generally the case in the area of toxicity prediction.) This is also reflected in public databases – in databases such as ChEMBL we have significantly more information on efficacy-related endpoints than on those related to safety.
One topic we left out of the discussion at this stage is metabolism – what actually happens to a compound in the body, and how can we anticipate effects not only of the original drug (the ‘parent compound’), but also its metabolites? Target-based activity profiling of course will not tell us anything about that, but empirical toxicity assays (or eg animal models) might, provided that they are metabolically competent in a way that translates to human metabolism. Likewise, even if toxicity occurs in animal models, this may be dose-dependent and only occur below therapeutic dose – and animal models may then provide biomarkers to follow in later clinical stages. So while there is still a potential problem it becomes far more manageable – at least we know what to measure, and what to look for.
Some of the recent computational challenges seem to contribute somewhat to an overly simplistic view on compound toxicity, say the Tox21 and CAMDA challenges, where if one is not careful this could be interpreted as a computational ‘label prediction problem’, where simply those win who get the better numbers from a computer. While those challenges have an honourable aim, toxic endpoints should in my opinion not be used without any attention to the biological context and in vivo relevance, since this is the situation the models will need to be used in. If e.g. the link of target activity to toxicity, or predictivity for novel chemical space due to focus only on overall model performance statistics, or PK/exposure or metabolism etc. are neglected then any computational model will simply have less practical relevance. This can then lead to claims that a certain level of ‘AI predictivity’ is achieved for toxic endpoints, but where applicability to a practical drug discovery situation is not always warranted to the same extent. It is of course easy to be carried away by technology, and I can understand this, but it would likely advance the field if such challenges could also eg involve practitioners in the (drug discovery and medical) field, so that the field is really advanced as a result. (Note: We are in touch with the CAMDA organizers exactly about this point and also participated in the challenge to exactly bring practical relevance to the table, which I believe is absolutely crucial to advance the field).
Of course there is not only the scientific question – sometimes it is about access to data, or legal barriers. When they are overcome then this makes clear, such as shown in recent work by Hartung et al., that computational models are very well able to make use of existing data, as shown in a study presenting Read-Across Structure Activity Relationship (RASARs) for a series of 9 different hazards caused by chemicals.
Interestingly, it can be seen that none of the above situations has ‘deep learning’ or AI as the solution (with the possibly exception of digital pathology, at least from the technical angle) – and even chemical space coverage is secondary, compared to the way of generating data for modelling, which needs to be appropriate for the problem in the first place.
I usually see this as ‘data > representation > algorithm’ – so an algorithm will not work if the representation is insufficient, and the representation will not solve problems with quantitatively insufficient data (or data unsuitable for the problem at hand) either.
The above are probably big problems, but somehow we need to proceed – so what can we do realistically in in silico toxicology?
The above discussion also gives us the answer of what we need to address in the different types of approaches – we need to work on extrapolating from model systems to the human situation in all of the above areas, meaning to improve our understanding of PK and exposure. In protein-based models an aim is to identify better where the predictive signal is; in biological readout (-omics) space the task is to identify relevant assay conditions for readouts and also the predictive signal for a given toxic endpoint. Empirical toxicity modeling requires a predictive assay endpoint in the first place, and then the model needs to be filled with sufficient data.
Some of the readout-to-toxicity links of course might come from current projects such as the Innovative Medicines Initiative (IMI) projects, eg eTox, eTRANSAFE and others. Let’s hope that the sharing of data, with as much annotation and on as large a scale as possible, and also where possible with academic/outside partners, will then help us find patterns in toxicity data better than in the past.
Currently much of the data we use in drug discovery is either sparse, noisy, or simply irrelevant to the problem at hand, and this article was aiming to describe the situation in the area of in silico toxicology, to provide a more realistic view on what is currently possible using ‘AI’ in this area. We are probably not limited by algorithms at this stage, we are limited by the data we have. This is not only due to the cost of generating data, it is partly due to more fundamental questions, such as translating exposure, and the question of how to generate data in a biological assay so that it can be used in different ways in the area, which is far from trivial (see section above on biological readouts). To address this will require working together, from the biological/toxicology/pharmacology domain to computer sciences and everything in between, and between industry, academia and government agencies – the better we understand what each other is doing, the better will be the outcome for everyone in the end for the field of predicting compound toxicity using computers.
I would like to conclude by saying that developing drugs is, of course, about balancing benefit and risk, so safety cannot be looked at in isolation. To use the example provided by Chris Swain (thanks Chris!) here: “Is a drug that relieves a patient from a lifetime of chronic pain, but increases the life-time risk of heart attack two-fold, of value”?
/Andreas
I would like to thank Graham Smith (AstraZeneca), Chris Swain and Anika Liu (both Cambridge) for input on this article, however all opinions expressed are solely my own.
P.S.: I have only realized after starting this article that the topic is very complex and very difficult to compress into a single blog post. I apologize for generalizations, and refer the reader to the reviews and articles cited above, and beyond, for more detailed information.
P.P.S.: I see it with amazement that, as a chemist, I am so much emphasizing the importance of biology these days. A drug has many angles though – the more angles we see a compound from, the better we can understand it.
Absolutely fantastic blog post Andreas! Looking much forward to see more!